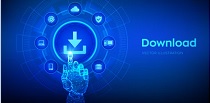

The combined search includes, but is not limited to, Journal of American Medical Informatics Association (JAMIA), Journal of American Biomedical Informatics (JBI), Nature Scientific Reports, PLoS One, ACM SIGKDD Conference on Knowledge Discovery and Data Mining (KDD), Neural Information Processing Systems (NIPS) and the Machine Learning for Health Care (MLHC) conference. We conducted a systematic review of deep learning studies using EHR data from PubMed and Google Scholar. This review summarizes the recent development of deep learning models for EHR data and suggests future research directions.

However EHR data also introduce many interesting modeling challenges for deep learning research. Second, large and complex datasets (eg., longitudinal event sequences and continuous monitoring data) are available in healthcare and enable training of complex deep learning models.
#Electronic medical records training northeast georgia manual#
First, for healthcare researchers, deep learning models yield better performance in many tasks than traditional machine learning methods and require less manual feature engineering. Interest in deep learning for healthcare has grown for two reasons. Over the past few years, an increasing body of literature confirmed the success of feature construction using deep learning methods (ie., models with multiple layers of neural networks). 2 It has changed the data analytic modeling paradigm from expert-driven feature engineering to data-driven feature construction. The resulting models often have limited generalizability across datasets or institutions.ĭeep learning has had a profound impact in many data analytic applications, such as speech recognition, image classification, computer vision, and natural language processing. Traditional health analytics modeling often depends on labor intensive efforts, such as expert-defined phenotyping 1 and ad-hoc feature engineering. However, it is challenging to create accurate analytic models from EHR data, because of data quality, data and label availability, and heterogeneity of data types. They consist of heterogeneous data elements, including patient demographic information, diagnoses, laboratory test results, medication prescriptions, clinical notes, and medical images. Electronic health record (EHR) data from millions of patients are now routinely collected across diverse healthcare institutions.
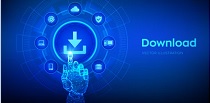